Advancing Your Advanced Analytics Capabilities
How to gain insights, efficiencies, and increase your organizational performance by adopting advanced analytics in an agile and lean manner.
As the amount of online traffic and connected devices has increased, so has the amount of data available.
And over time, this unprecedented influx of information—along with massive advances in storage and computing technology—has opened the door for much more advanced analytics, radically changing how enterprises make decisions and gain a competitive edge.
Putting advanced analytics to work is not a quick and easy process, however.
Storing data, sorting it, finding the right projects to build your advanced analytics capabilities—each of these is a step in a journey.
On this page, you’ll find information on the right path to take in deploying advanced analytics for your organization
Page Contents:
Click a link to jump to that section
What is advanced analytics?
Analytics is applying a logical method to solve a business problem using analysis and data to produce value and impact.
Organizations grapple with strategic business problems like cost-cutting and innovation. Initiatives to solve these problems tend to stress budgets and organizational structures. Data analytics can be a powerful tool to alleviate these issues when used correctly.
The right analytics approach is an informed solution backed by the correct data, process, methodologies, technology, and insights. Not just insights, but actionable insights—the kind that allows for easy decision-making, enables new thought processes, and invokes positive action by deploying data-led, hypothesis-driven decisions backed by intuition and vision.
Any description of advanced analytics needs to begin with the catch-all term “Big Data.” Large amounts of structure and unstructured information that can be captured from a wide range of resources.
Most enterprises are now utilizing this data in some form or fashion, even if they don’t label it as such. Purchase records, website clicks and page views, location and demographics information, device usage—each counts as a piece that can be stored, refined, and analyzed.
The availability of all this data has changed how enterprises can leverage information to improve their business. Instead of simply being reactive using data, organizations can now employ progressive technologies such as:
Predictive analytics
In contrast to traditional business analytics, predictive analytics mines vast quantities of information in order to glean projections. These projections are more than just educated guesses—in fact, they can assist enterprises in:
- Optimizing marketing initiatives
- Streamlining supply chains
- Forecasting sales trends
- Managing potential logistical challenges
Artificial intelligence
Once relegated to sci-fi novels, artificial intelligence (AI) is now a tool enterprises are putting to work in a number of different ways, including:
- Producing efficiencies in internal operations
- Automating repetitive tasks
- Utilizing chatbots for customer service
- Optimizing shipping logistics
Machine learning
If AI is how analytics are put to work, machine learning (ML) is a powerful tool to derive lessons from those analytics. In general, ML can be broken down into:
Supervised learning uses clearly defined input to train a model that can be used to give an answer to a given question. For example, if you want to predict how many people will cancel cable in the coming year, you can define example inputs that are known (cancellations) and train the model on labeled historical data to eventually arrive at a model that can accurately predict the outcome for new data.
Unsupervised learning is used when you want to find inherent relationships from the data, such as customers who buy one product also buy some other product. The data isn’t as clearly defined. Instead, you’re essentially use the ML model on unlabeled data to extract patterns from it.
Examples of industries already leveraging ML are:
Finance and healthcare, where models are used to predict possible cases of fraud
Retail, where companies are utilizing the technology to greatly improve product recommendations.
Use cases for advanced analytics
Now that we’ve talked about what advanced analytics is and why it’s important, let’s look at three examples of it being put to use. One in finance, one in healthcare, and one in retail.
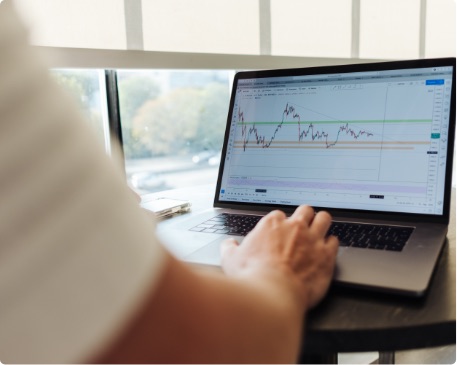
Finance
Every minute of every day, millions of credit cards are being swiped around the world. In order to identify potential cases of fraud, credit card providers deploy ML models to help determine irregularities based on previous purchasing habits, locations of purchases, even times of day purchases are made.
Using the output of these models, credit card providers are able to know when a customer has had their information stolen before the customer does and can take preventative measures — all without slowing down the flow of transactions.
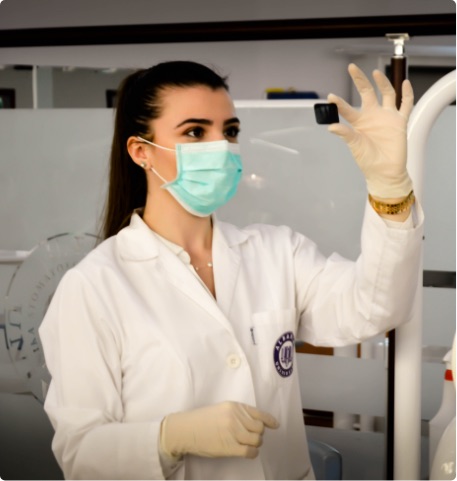
Healthcare
Redapt client Zelis is a healthcare technology company that, among other things, processes out-of-network claims between doctors and insurance providers. Given the sheer amount of claims, the company needed the ability to predict and isolate claims that were potentially fraud, waste, or abuse (FWA).
To help them with this problem, Redapt built out a framework for the company to efficiently sift through data to identify and fingerprint historic costs related to procedures. This framework was flexible enough to be modified with new information as it arrived.
By leveraging the public cloud, Zelis’ data scientists were able to run advanced analytics models to not only flag potential FWA claims, but accelerate the processing of non-fraudulent ones.
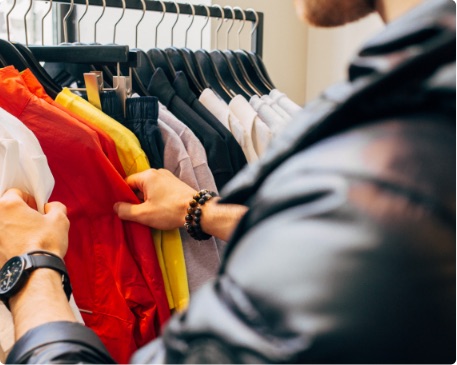
Retail
Online personal styling service Stitch Fix connects customers to clothes by utilizing advanced analytics to develop algorithms based on popular combinations and styles.
These algorithms then intelligently style entire outfits based on customer interests, seasonality, fit, and so much more. This way, all customers have to do is simply tell the company a little about their likes and dislikes. Then, before they know it, they’ll receive outfits based on that information.
The benefits of this approach are twofold:
- Stitch Fix is able to serve more customers than traditional, in-person styling services.
- Customers are able to have an experience normally reserved for the more affluent.
Advanced analytics trends
According to The Enterprisers Project, advanced analytics started taking center stage in 2019. In the coming years, its usage is only going to grow as more and more enterprises adopt the cloud and develop data strategies for technology such as high-performing AI.
Similarly, the popularity of ML will continue to increase, particularly for processes like IT Operations Analytics (ITOA), where models can help identify IT issues faster and, after an issue is identified, discern the cause and effect in a matter of hours rather than days.
On the customer-facing front, AI chatbots and ML-based product recommendations will only get more effective and granular. And, as major retailers look to better optimize shipping logistics and cut down their carbon footprints, advanced analytics will chart the way.
Another key driver of this kind of analytics going forward will be its continued increase in accessibility as the tools available become more affordable.
From startups to traditional mom and pop stores, companies of all sizes in coming years will be able to leverage insights—not just descriptive analytics (what has happened) but diagnostic (why it happened) and predictive (what will happen) analytics as well.
The most common challenges in adopting advanced analytics
It has been estimated that nearly 90% of advanced analytics projects like ML fail to reach production.
One of the common driving factors behind this failure rate is the disconnect between IT and data science.
IT tends to stay focused on making things available and stable. They want uptime at all costs. Data scientists, on the other hand, are focused on iteration and experimentation. They want flexibility and performance.
Beyond internal disconnects, many of the roadblocks Redapt has seen enterprises encounter on the way to adopting advanced analytics come down to data. Specifically:
Traditional organizational power structures
In reality, technology is hardly ever the core blocker to transformative analytical change. Most of the friction comes from antiquated organization parent-child structures that make cross-functional and agile analytical transformation almost impossible to implement effectively.
Data quality
Results from AI and ML models are only as good as the data used to arrive at them. Without proper data processing and pipelines, actionable data will be hard to come by and your models will fail.
Data organization
AI and ML need to know where to look within data sets. A failure to properly organize data with sourcing and tagging will only send advanced analytics models into a sea of information with no sense of direction.
Data storage
Advanced analytics requires access to a substantial amount of data to be effective. While the public cloud has made storing the necessary information more cost-effective, if your organization relies on cloud-native tech stacks on premises or at a co-location, you can easily misjudge the amount of storage you need.
Data governance and security
The more data you make available throughout your organization, the more business objectives you are able to achieve.
This availability requires following proper governance over your data in order to keep it safe and ensure only specific data is available to specific teams that need it. The challenge, however, is putting governance rules in place that every team in your organization follows.
Levels of advanced analytics performance
While every organization working to leverage advanced analytics begins at a different starting position, there are generally four different levels they pass through on their journey to the elite performance that generates better decisions for their businesses.
At this base level, your organization has yet to tap into the full potential of your data. You have limited live data available, lack tooling to execute analytics, and are not able to conduct reporting on your data.
While members of your leadership team have likely expressed interest in adopting advanced analytics, there’s been no firm commitment.
You’ve made the commitment to building out your advanced analytics capabilities and have aspirations to centralize data access within your organization.
Still, your data analysis is still happening in a vacuum, and while you’re able to conduct trend analysis and have data classification and management solutions in place, your insight stemming from siloed data is still limited.
You have completed data value definitions and are blending resources for your analytics and engineering teams.
In addition, you have all the resources available to achieve the required performance of your models, you are leveraging cloud data services, and governance has been automated and is actively monitored. Because of this, you are able to make ML-driven business decisions.
You have achieved elite performance with your advanced analytics and are making data-driven decisions throughout your organization.
Automation is reducing your analytics costs and you’re able to easily test models against data. You are also able to increase your efficiency by identifying historical trends, you have improved your forecasting accuracy, and you are utilizing AI to enhance your customer interactions.
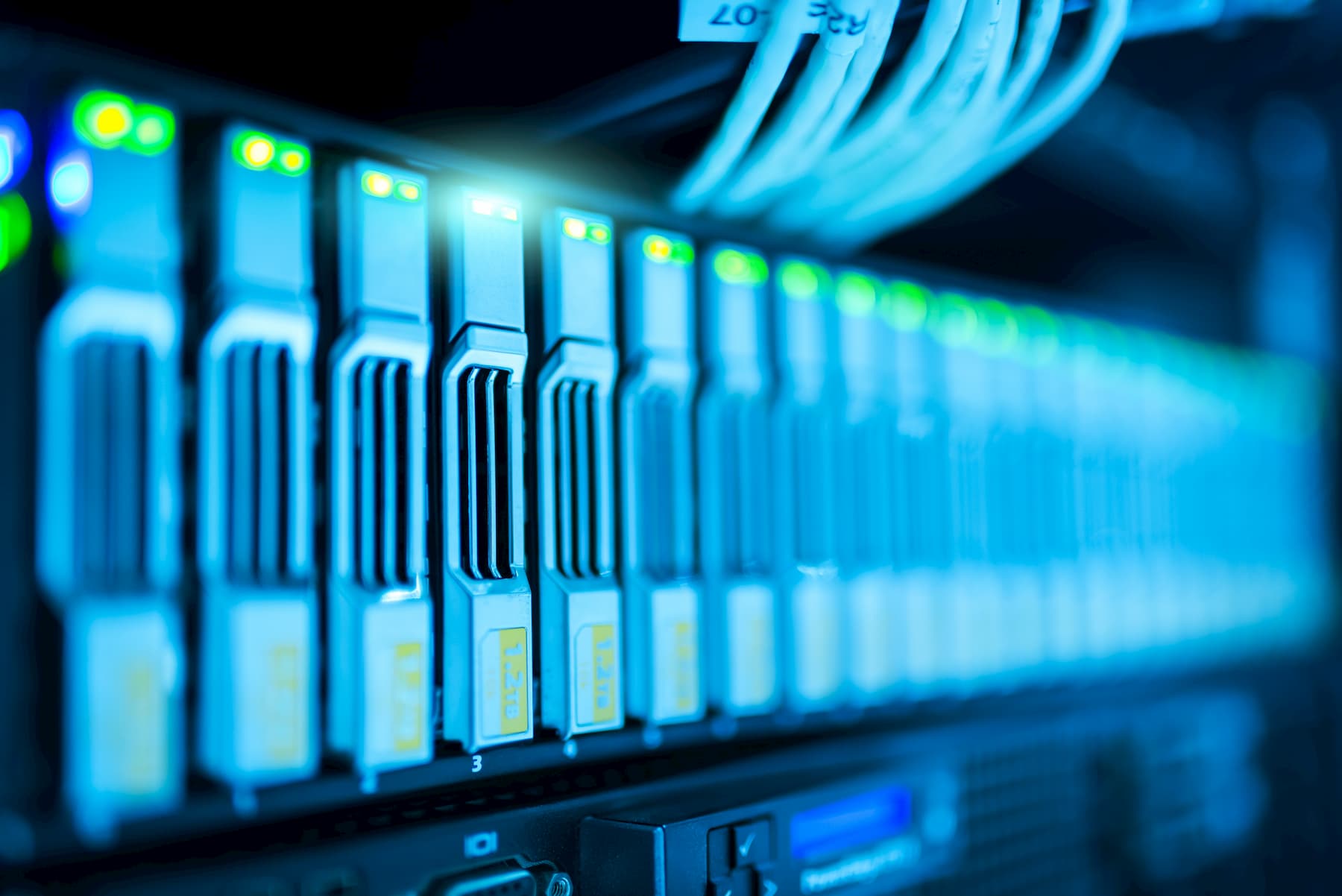
Guide
To start getting strategic with your data …
... as well as learn everything you need to know about building a modern datacenter, download our white paper A Strategic Approach to Data Storage.
Download Free White PaperThe path to advanced analytics
Redapt recommends several high-level, key areas and objectives to focus on as you start the process of adopting advanced analytics.
Embracing a cross-functional agile analytics organizational paradigm
Break down silos across the organization by following successful self-management techniques like Holacracy that allow for true inter-departmental collaboration and experimentation.
Conducting a data assessment
to provide you with a holistic view of all your data and where it’s arriving from. This process involves combing through all your current analytics workloads and sources, examining the completeness and value of your data, and identifying which of your teams need access to specific data sets.
Simplifying your data
by overhauling the way your data is distributed and queries are handled—scaling out vs. scaling up. This involves spreading your data in such a way that valuable information is not buried beneath a mountain of useless information.
Warehousing your data
from varied sources into a centralized location. This data can arrive structured, semi-structured, or unstructured, and the warehouse is where the information is transformed and processed.
Data warehousing also makes it possible for:
- Users to easily access critical data from a wide range of sources quickly
- Turnaround on analysis and reporting to be greatly accelerated
- Security and compliance to be applied across your data
By determining the answers to these questions, you’ll have a better understanding of what you’ll be able to achieve in the cloud as well as those areas in your current code development workflow that need to be overhauled.
Democratizing your data
to free it from traditional IT gatekeepers so it is accessible throughout your organization—from analytics and executives, to marketing departments. In addition, you will want to employ technologies like:
- Data virtualization to pull in and manipulate data regardless of data inconsistencies or file formats
- Data federation to aggregate information into a single virtual database using metadata
- The cloud to centrally locate your data and partition it out into governed data lakes for access
In a nutshell …
Advanced analytics are going to be an ever-growing force for enterprises going forward. Tools like predictive analytics, AI, and ML will drive the creation of better products and services, streamlined operations, and very real, competitive advantages for companies.
Successfully putting advanced analytics to work requires a lot more than simply throwing algorithms into a mass of data, however. So before you take the plunge, you need to understand:
- Your current capabilities as an organization
- What data you have access to
- What you’re trying to achieve through advanced analytics
- The technologies and infrastructure you need to have in place in order to successfully utilize advanced analytics
If your organization is ready to adopt advanced analytics, we can help you through every step of the process, including:
- Assessing your current capabilities to gauge your readiness to adopt AI
- Capacity planning to ensure you are able to manage high-performance AI workloads without disrupting your other initiatives
- Infrastructure design, build, and deployment so you can start leveraging unstructured data throughout your organization
Get in touch with a Redapt expert to get started.
Success stories
Case Study
Streamlining Security Operations with SUSE Rancher Deployment at a Cybersecurity Company
Case Study
Smarter Data Informed Decisions
Related resources
Guide
The Executive's Guide to AI and ML Adoption
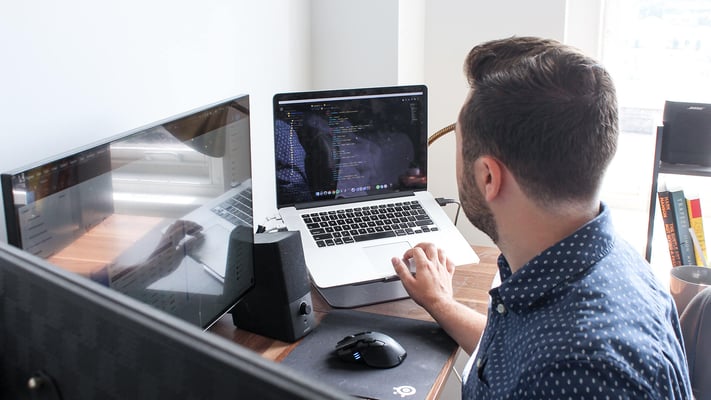
Blog Post
How to Get More from Data Science
The flood of data now available to enterprises is fueling a revolution in data science.
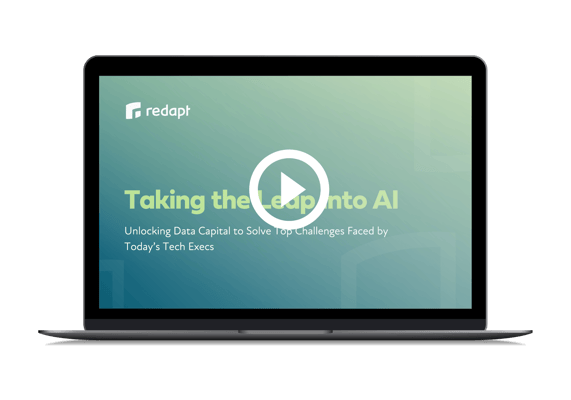
Blog Post
Watch the Webinar: Taking the Leap into AI
In this webinar, Redapt experts Amie Mason and Bryan Gilcrease discuss how your organization can unlock its data capital and adopt AI technology.