Accelerating Your Success with Artificial Intelligence and Machine Learning
How to overcome challenges and achieve success in building out your advanced analytics capabilities
Thriving businesses require faster time to insight and the agility to implement insights across the organization.
Artificial Intelligence (AI) and Machine Learning (ML) are increasingly valuable tools for accelerating insight. But in order to use them effectively, especially at scale, your objectives, data strategy, data scientists, and IT must be aligned.
Getting your AI/ML pipeline and infrastructure in place can be complex. For example, creating deep learning models that can identify things like fraudulent insurance transactions requires faster storage and networking. These, in turn, require more power and cooling and introduce unique challenges for implementation and operations.
On this page, you will find everything you need to know about creating an enterprise infrastructure capable of powering advanced analytics capabilities like AI and ML. You’ll also learn how making the investment now will help you unlock new opportunities, make smarter decisions, and gain a competitive advantage down the road.
The growing impact of AI and ML on business
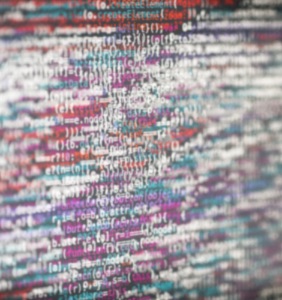
The ML revolution is being powered by the rise of unstructured data.
Every day, new technologies and devices are coming online, each producing information that can be mined for insights, used to identify potential efficiencies, to accelerate innovation, and to provide better customer service. More and more, ML is being used outside the scope of the IT teams as key executives are learning to use it to speed up innovation.
The four most common motivations enterprises have for adopting AI and ML are:
Data analysis
to make smarter business decisions, predict customer wants and needs, create new products, and identify potential issues or instances of fraud
Internal communications
such as booking direct and cost-effective travel, managing vacation hours, and making better use of resources
Automation of tasks
like data entry, basic natural processing, and the updating of records
Customer service
via intelligent chatbots that can answer questions while reducing the need for always available support teams
Examples of industries impacted by ML
If AI is about putting data analytics to work, ML is about training algorithms to make decisions.
This training requires a substantial amount of data—so much data, in fact, that storing it and then applying analytics and ML models to the amount needed was outside the budgets of most enterprises until the public cloud arrived.
As more and more enterprises have adopted the cloud or hybrid platforms, though, usage of ML has ramped up. For an idea about how it’s being used, let’s look at three industries: healthcare, finance, and retail.
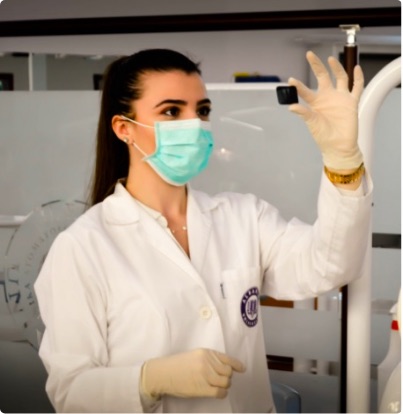
Healthcare
Americans spend trillions of dollars on healthcare each year. With that amount of money moving around, the industry is ripe for fraud.
Through ML, healthcare providers and insurance companies are able to repeatedly run models in a sea of data. They are then able to apply the learning from those models to build out systems to flag potential fraudulent activity.
On a more positive note, ML also helps healthcare researchers predict things such as specific regions that may be susceptible to particular strands of flu, or areas where unhealthy pollutants will increase in the environment.
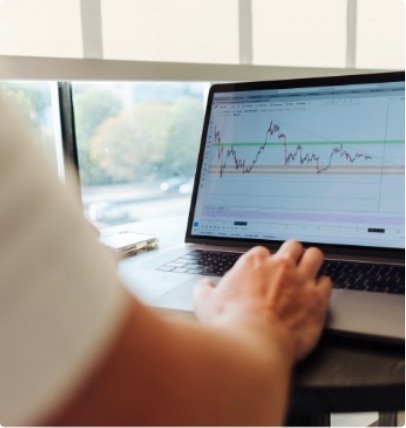
Finance
Given the millions of credit card transactions that happen around the world on a minute-by-minute basis, keeping up with potential errors or crimes such as identity theft means being able to identify and address problems close to instantly.
ML models can arm credit card providers with the ability to determine irregularities based on things like a customer’s previous purchasing habits, locations of purchases, and even times of day purchases are made.
As a result, it’s often possible for credit card companies to realize a customer’s information has been stolen and take preventative measures before the customer has—and they can do it without slowing down the flow of legitimate transactions.
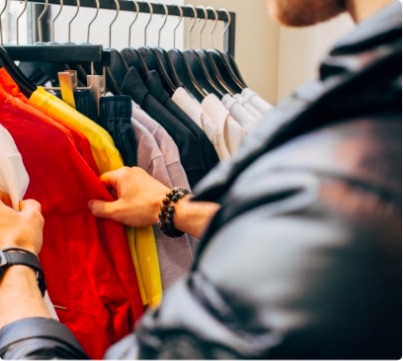
Retail
Online clothing retailers such as Stitch Fix are using ML models to develop popular combinations of clothing based on data. They then are able to provide customers with outfits based on their interests, seasonality, fit, and more.
Not only does this deliver more choices to customers, it also helps the company bring personal styling service—traditionally a time-consuming process reserved for higher income customers—to a much wider customer base.
Overcoming obstacles to AI and ML
It has been estimated that approximately 90% of ML models dreamed up by data scientists never actually make it into production.
While the percentage for AI initiatives is likely lower, many enterprises still encounter roadblocks on their way to putting the technology to work. The leading causes of this problem are twofold:
- IT teams are often unfamiliar with the software and specialized hardware necessary to deploy AI and ML models.
- More pervasive cause, is a disconnect between IT and data science. For the most part, IT tends to focus on making things available and stable, while data scientists like to experiment and break things.
While solutions such as Kubeflow exist to help enterprises navigate software and hardware problems, getting IT and data scientists on the same page takes a more holistic approach. This, again, is where understanding your technical maturity before attempting to adopt AI and ML can be critical.
With a thorough assessment of technical maturity, an enterprise can come to agreement on:
- What they are trying to achieve with AI and ML
- How they can address competing business priorities
- A comprehensive roadmap to AI and ML adoption
In other words, IT and data scientists can enter the process on the same page, with each department knowing their role and what their counterparts need to accomplish the enterprise’s goals.
Roadblocks to AI and ML success
While the above are often the desired outcomes of AI and ML adoption, getting to those outcomes is often a challenge for most businesses due to some common roadblocks. There are a number of reasons organizations fail at adopting AI and ML. These include failures in infrastructure, lack of skill sets, and more. Specifically, we’ve found that a number of businesses we’ve worked with experienced some mix of the following challenges:- Data quality
- Data organization
- Technical capabilities
- Storage issues
Data quality
Results from AI and ML are only as good as the data that is used to produce them. Without having the infrastructure in place to properly assist in the processing of data, actionable information will be hard to come by, findings can be conflicting, and AI and ML models will fail.
Data organization
Similar to data quality, AI and ML need to be able to actually know where to look within large quantities of information. A failure to properly organize data with sourcing and tagging will only send models into a sea of information with no sense of direction.
Technical capabilities
Compared to traditional software engineering, AI and ML development require new roles and processes. Data scientists begin by experimenting to find the right combination of data and algorithms to develop a model, and this step is new and often falls outside the traditional IT organization. There are further complications with ensuring governance and security of data, as well as controlling storage and compute costs.
Storage issues
AL and ML require a great amount of data in order to be effective.
The public cloud has made storing the necessary information more cost-effective. However, many enterprises relying on on-premises datacenter infrastructure can easily misjudge the amount of data storage they need as part of their infrastructure.
Levels of AI and ML performance
While every organization’s path to AI and ML adoption begins from a different starting position, there are generally four different levels that need to be reached in order to fully leverage the potential of advanced analytics.
If your organization is at this level, you are currently conducting little reporting on your data and underestimate its potential.
At the same time, you are interested in what you can achieve by adopting AI and ML but have questions about how your organization can get to the point where the tools will be effective and worth the investment.
You have centralized data access, classification, and management.
Tests on data are being conducted manually and you are able to do things like trend analysis. But your business insights are still limited due to your data analysis happening in a vacuum.
At this level, your organization fully knows the value of your data.
Your teams have the ability to quickly explore tests and leverage cloud data services, and you have implemented data governance with best practices. All the resources are in place to help you achieve the required performance and you are able to deliver business-driven ML insights.
You are at the peak level of AI and ML utilization.
You can employ predictive analytics on live data and accurately forecast results. In addition, you are utilizing dynamic dashboards and are creating automatic actions based on analysis.
At this level, your business is being positively impacted by AI and ML due to your ability to make smarter decisions faster than your competitors while delivering to your customers innovative new products informed by data.
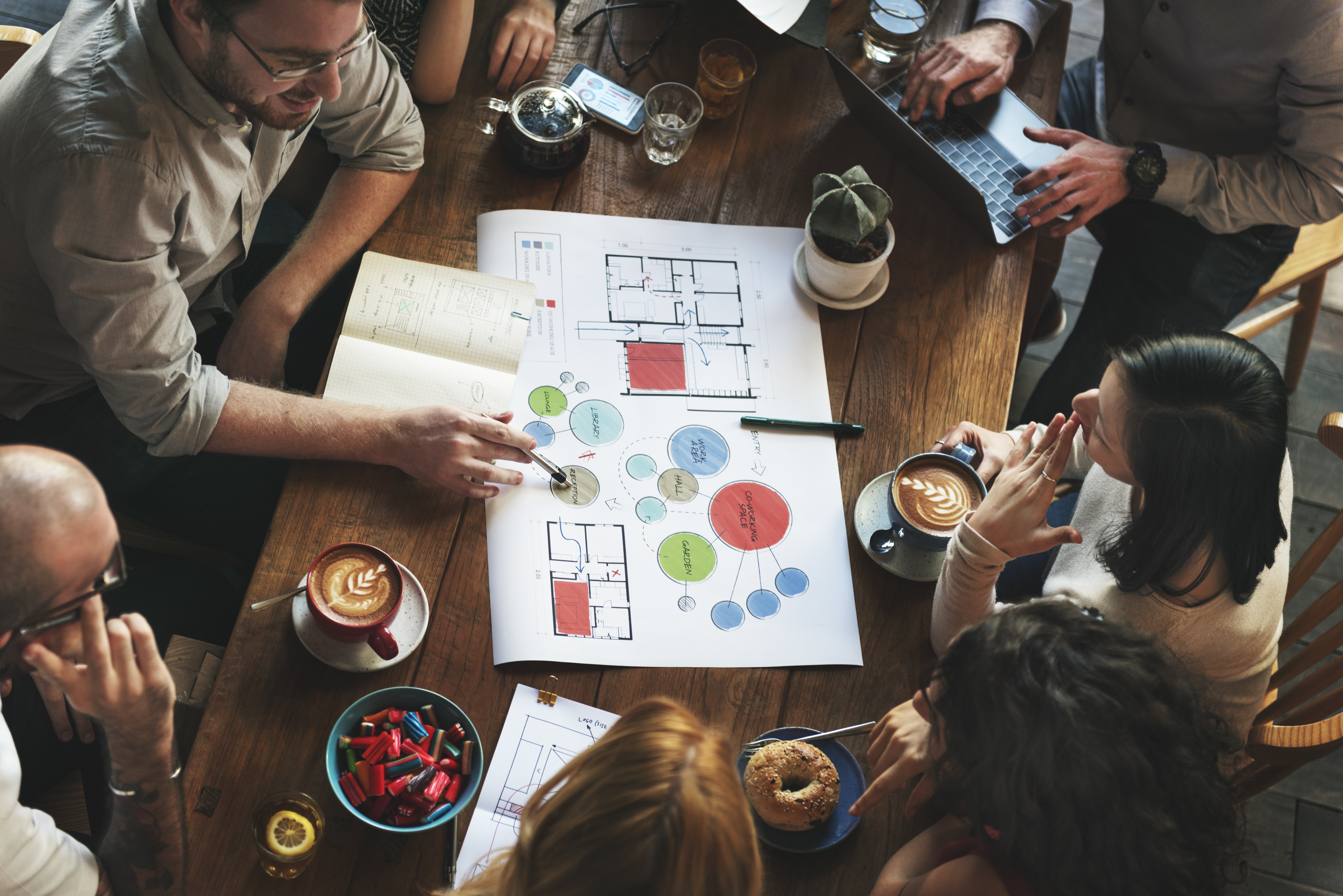
eBook
Kick-Off The AI Adoption Process Today
Our free eBook walks you through the three steps you need to take to successfully bring AI solutions to your enterprise.
Download Free eBookThe path to adopting AI and ML
At a high level, getting your organization in a place where it is ready to adopt AI and ML should follow this process:
Identify a use case
Evaluate your business, industry, and competitors to find the right ideas to try.
To build a business case, search for existing algorithms and opportunities for incremental improvement to base your model on, then plan your roadmap of experimentation. Your first AI and ML projects should be at a scale that is achievable and will make an immediate and tangible impact.
Build a plan and focus on your infrastructure
Decide how your teams should implement your initial project and where specific workloads will run.
Build out your infrastructure so data is centrally hosted and easily accessible. Many times, the best solution is a mixture of public cloud and on premises datacenters depending on legal requirements, performance needs, and ROI. In fact, high performance hardware like GPUs, low latency networking, and all flash storage can be cheaper long term and provide better performance when hosting workloads on-premises.
Clean and manage your data
Identify which of your data sets are suitable for AI or ML workloads, then analyze those sets to determine what information is valuable and what can be discarded.
To cleanse your data, set up scripts or workflows to standardize the flow of incoming data. You will also need to locate gaps in your data and, if need be, work with third party data sources to fill those gaps.
Your goal is to provide your data scientists and IT teams with a central repository of data that has sources of truth and is governed to ensure access to only those who need it.
Finally, if need be, find the right partner
Most enterprises adopting AI and ML will quickly recognize that they need help. While bringing in talent is always a possibility, working with a partner is often a more cost-effective way to accelerate results.
When looking for a partner, make sure they are able to:
- Help you identify the right project to begin with
- Recommend the hardware and infrastructure solutions to get the most out of AI and ML without overprovisioning and overspending
- Walk you through and implement the cleansing and management of your data
- Train you in how best to take your AI and ML models from development to production.
In a nutshell …
With an unprecedented amount of data now available, enterprises are utilizing advanced tools like AI and ML to make smarter decisions, create innovative new products, and deliver better service to customers.
Successfully adopting AI and ML can be a challenge, but by focusing on what you’re trying to achieve with the tools, and the infrastructure and skills you need in place to get there, it can be done.
For a solution to kickstart your AI/ML journey, check out our ML Accelerator program to learn how you can go from zero capabilities to bringing your own advanced analytics models into production quickly.
And to learn more about AI and ML in general, contact one of our experts today.
Success stories
Case Study
Streamlining Security Operations with SUSE Rancher Deployment at a Cybersecurity Company
Case Study
Smarter Data Informed Decisions
Related resources
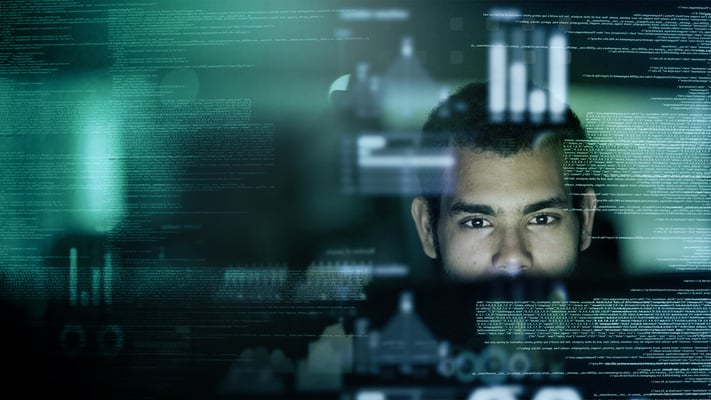
Blog Post
Navigating Technological Challenges: How Redapt Can Drive Your Success
Businesses must learn to leverage the latest technology to maintain a competitive advantage … or let the competition win.
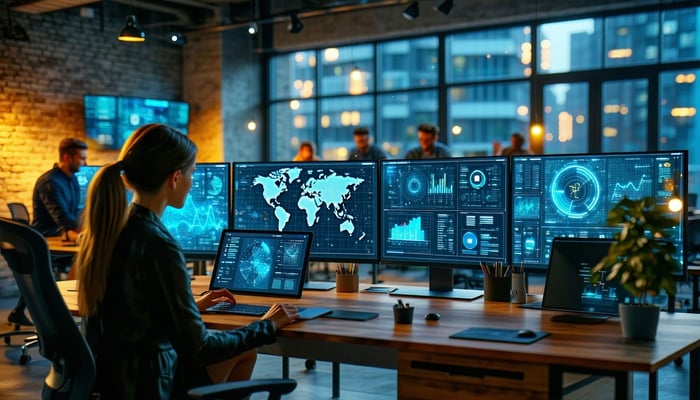
Blog Post
Leveraging AI for Semantic Understanding and Risk Mitigation
Data is the lifeblood of modern enterprises. Yet, the sheer volume and complexity of unstructured data many organizations deal with has resulted in chaos—a labyrinth of potential risks and hidden...
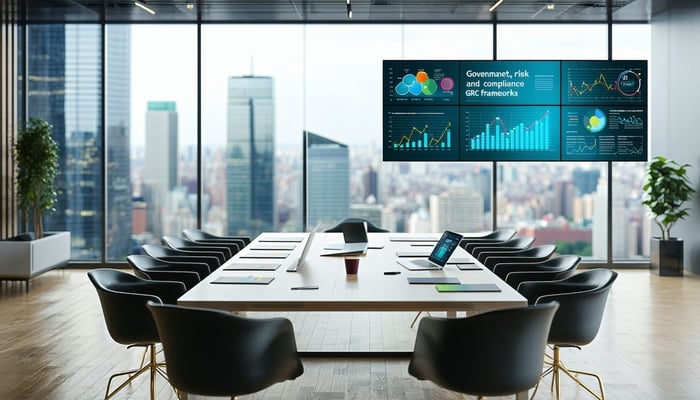
Blog Post
Unlocking Business Potential with GRC Frameworks
Businesses today are navigating an increasingly complex landscape. Rapid technological advancements, mounting cyber threats, and evolving regulations have created a perfect storm of challenges. Even...